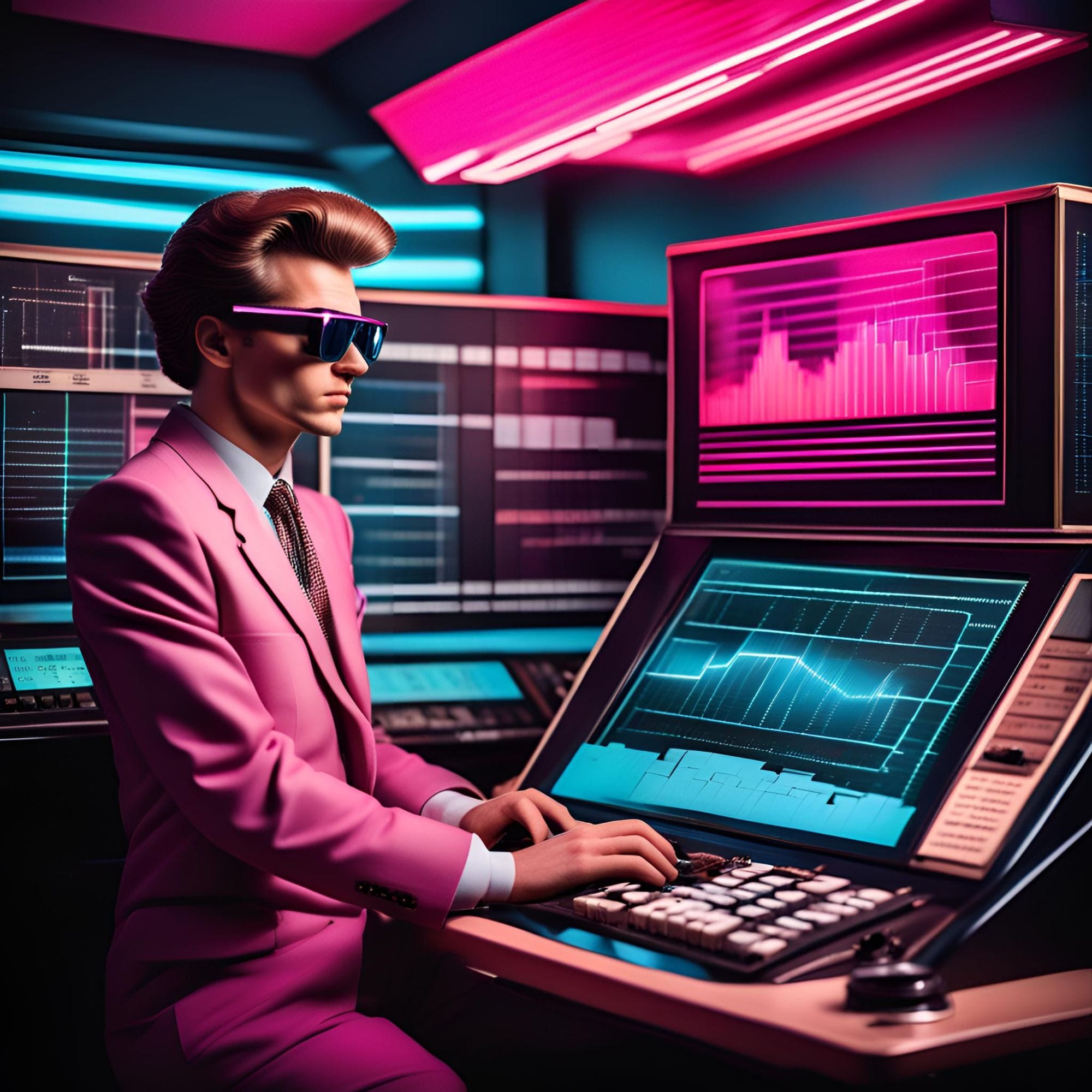
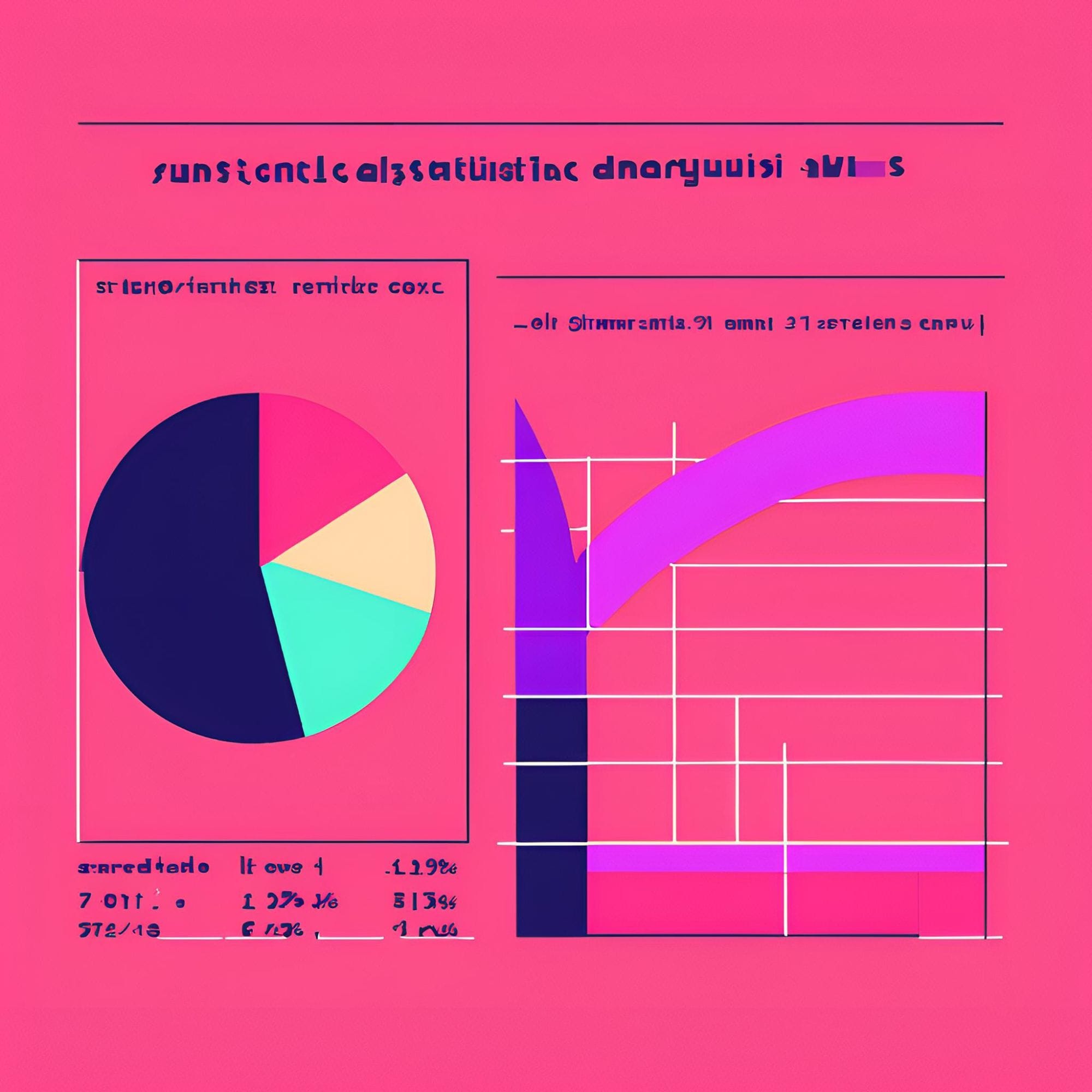
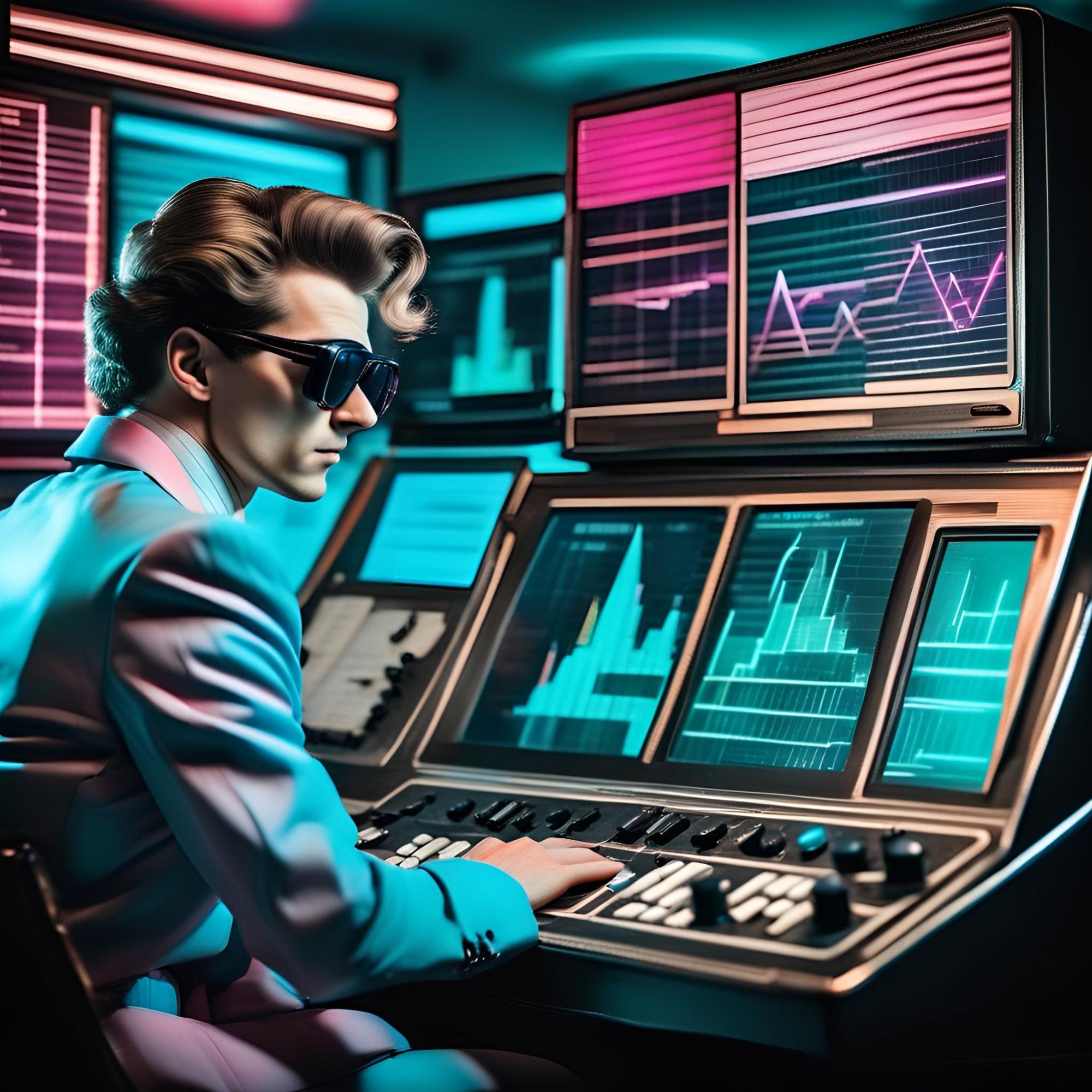
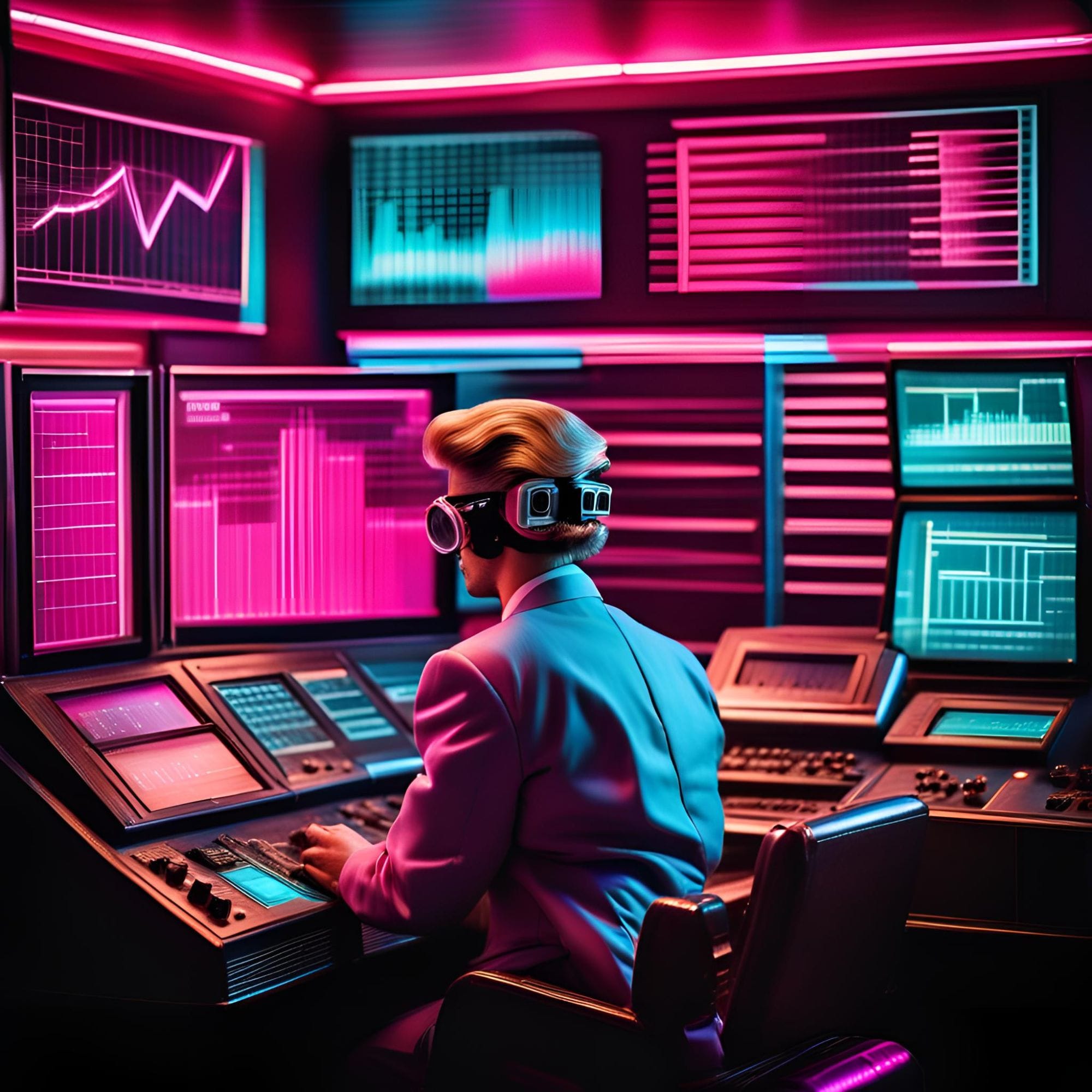
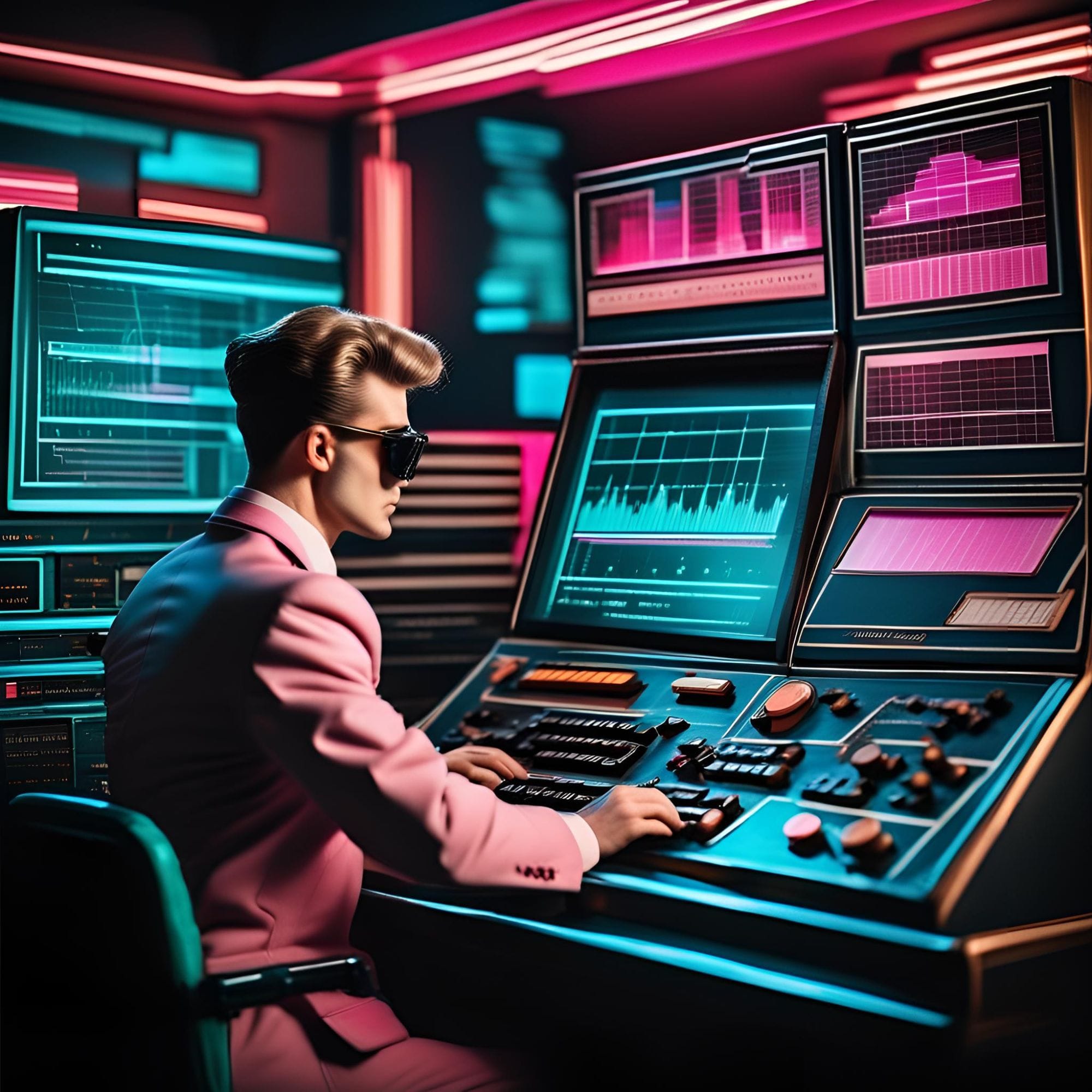
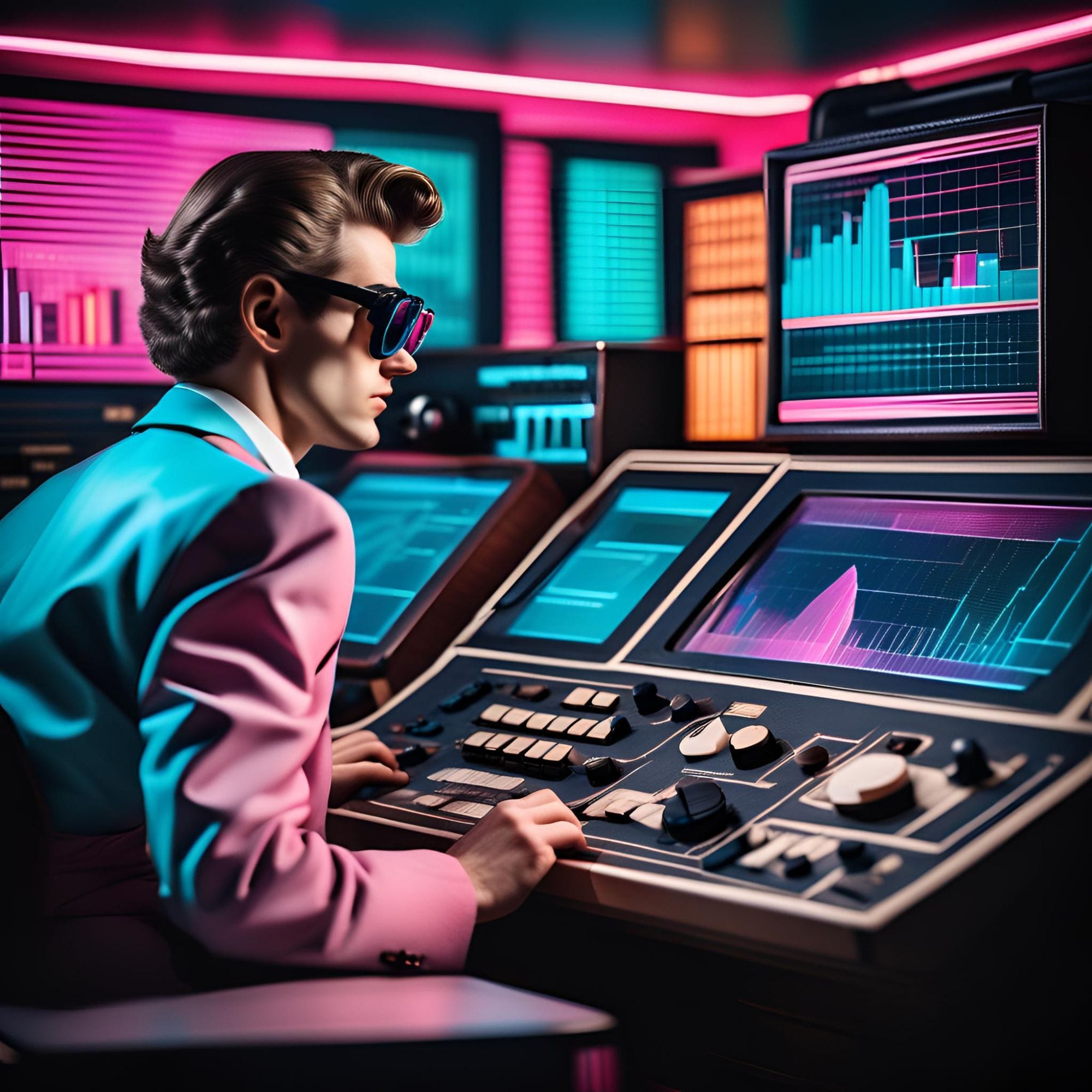
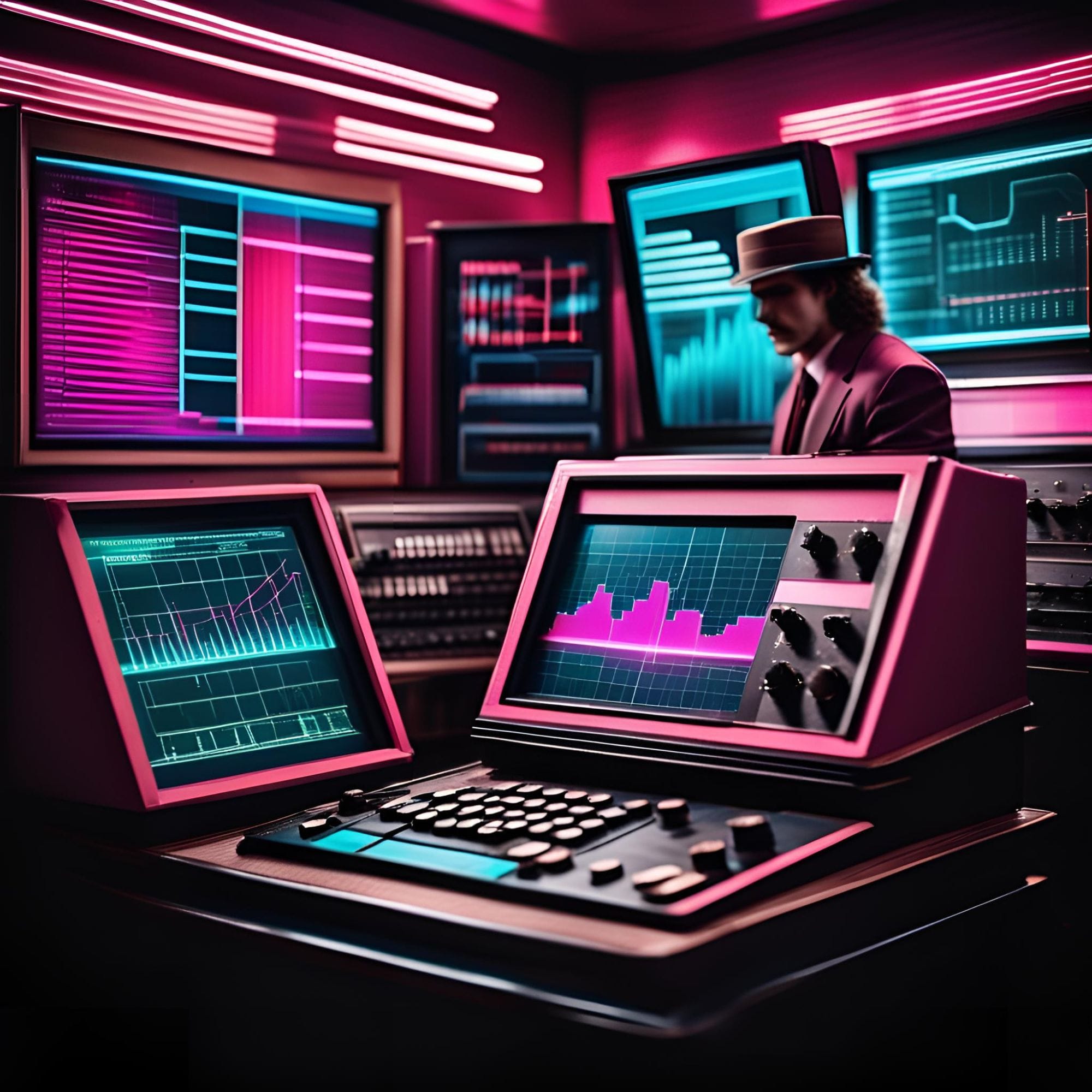
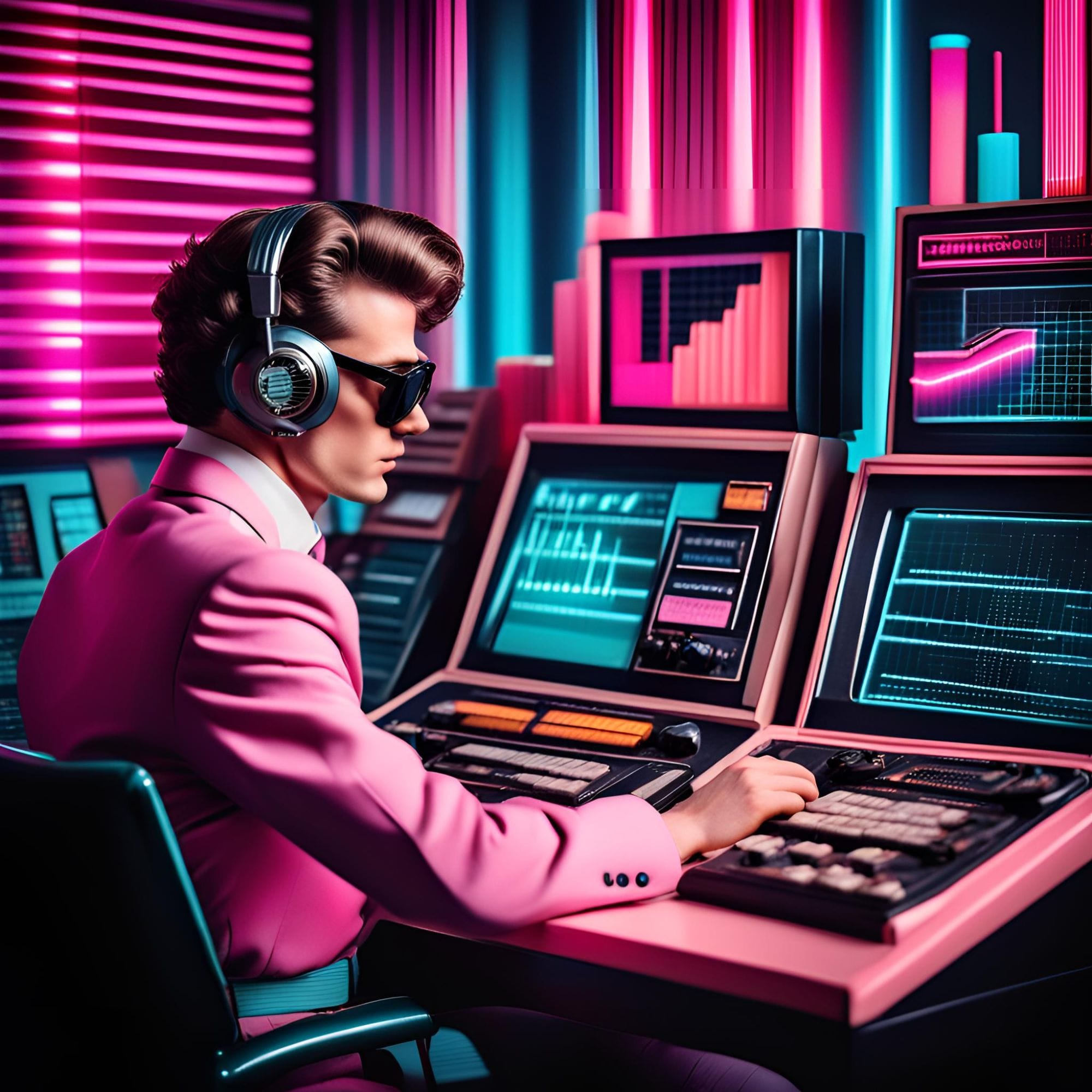
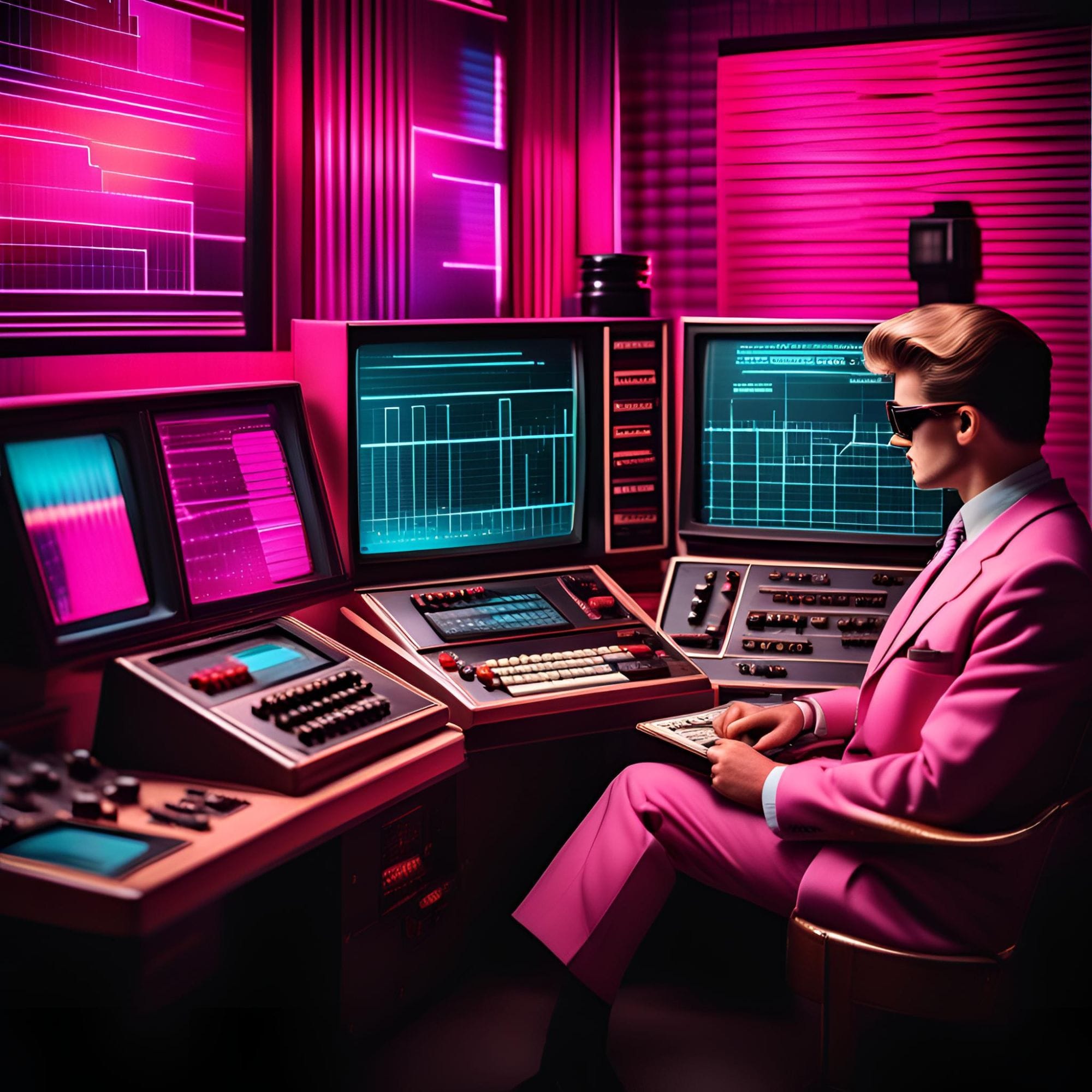
buymeacoffee.com coming soon.
Introduction
Statistical analysis is a powerful tool used in various fields to make sense of data, draw conclusions, and make informed decisions. By applying mathematical principles and methods, statisticians can uncover patterns, trends, and relationships within datasets that might not be immediately apparent. In this article, we will delve into the fundamental concepts of statistical analysis and explore the significance of confidence in drawing accurate conclusions from data.
Confidence in statistical analysis is crucial because it allows us to quantify the reliability of our results. It provides a measure of how certain we can be that our findings are representative of the larger population or phenomena being studied. Without confidence, the conclusions drawn from statistical analyses may be unreliable or misleading.
The purpose of this article is to provide a concise overview of statistical analysis, focusing on key concepts such as mean, median, mode, standard deviation, variance, hypothesis testing, confidence intervals, margin of error, and significance levels. By the end of this article, readers will have a solid foundation in the principles of statistical analysis and an understanding of how confidence plays a critical role in the process.
Let's explore the world of statistical analysis and learn how to navigate it with confidence.
Key Concepts in Statistical Analysis
Statistical analysis is a powerful tool used to make sense of data and draw meaningful conclusions. In this section, we will delve into some key concepts that form the foundation of statistical analysis.
Mean, Median, and Mode
The mean, median, and mode are measures of central tendency that help us understand the typical or average value in a dataset. The mean is calculated by adding up all the values in a dataset and dividing by the total number of values. The median is the middle value when the data is arranged in order, and the mode is the value that appears most frequently.
Standard Deviation and Variance
Standard deviation and variance are measures of dispersion that indicate how spread out the values in a dataset are. The standard deviation is the square root of the variance, and both provide insights into the variability of the data points around the mean. A low standard deviation or variance indicates that the data points are close to the mean, while a high standard deviation or variance suggests greater variability.
Hypothesis Testing
Hypothesis testing is a fundamental concept in statistical analysis used to make inferences about a population based on sample data. It involves formulating a null hypothesis (no effect or no difference) and an alternative hypothesis (an effect or difference exists) and using statistical methods to determine the likelihood of observing the results if the null hypothesis is true.
Understanding these key concepts in statistical analysis is essential for conducting meaningful analyses and drawing valid conclusions from data. In the next section, we will explore methods to establish confidence in our statistical analyses to ensure the reliability of our findings.
Methods to Establish Confidence in Statistical Analysis
Statistical analysis is a powerful tool that allows us to draw meaningful conclusions from data. However, it is crucial to have confidence in the results obtained through this analysis. In this section, we will explore key methods that help establish confidence in statistical analysis.
Confidence Intervals
Confidence intervals provide a range of values within which we can be confident that the true value of a parameter lies. When we calculate a confidence interval, we are essentially estimating the range in which the population parameter is likely to fall. For example, if we calculate a 95% confidence interval for the mean, we are saying that we are 95% confident that the true population mean falls within that interval. The width of the confidence interval is affected by factors such as sample size and variability in the data.
Margin of Error
The margin of error is a measure of the accuracy of an estimate. It quantifies the range within which the true value is likely to fall. A smaller margin of error indicates a more precise estimate, while a larger margin of error suggests greater uncertainty. By understanding the margin of error associated with a statistical estimate, we can gauge the reliability of the results and make informed decisions based on the data.
Significance Levels
Significance levels play a critical role in hypothesis testing, a key component of statistical analysis. The significance level, often denoted as alpha (α), represents the probability of rejecting the null hypothesis when it is actually true. Common significance levels include 0.05 and 0.01, indicating a 5% and 1% chance, respectively, of making a Type I error (incorrectly rejecting a true null hypothesis). By setting an appropriate significance level, researchers can control the risk of drawing erroneous conclusions from their data.
By utilizing these methods to establish confidence in statistical analysis, researchers and practitioners can ensure the reliability and validity of their findings. Confidence intervals, margin of error, and significance levels provide a framework for interpreting results and making informed decisions based on data-driven insights.
Conclusion
In conclusion, statistical analysis serves as a powerful tool for deriving meaningful insights from data, guiding decision-making processes, and drawing reliable conclusions about the population from which the data is derived. Throughout this article, we have delved into the essential concepts that underpin statistical analysis and the critical role that confidence plays in ensuring the validity and reliability of our conclusions.
By exploring key concepts such as mean, median, mode, standard deviation, variance, and hypothesis testing, we have laid the groundwork for understanding how data is analyzed and interpreted in statistical studies. These concepts provide the foundation upon which more advanced statistical techniques and methodologies are built.
Moreover, we have discussed methods to establish confidence in statistical analysis, including the use of confidence intervals, margin of error, and significance levels. These tools enable researchers and analysts to quantify the uncertainty inherent in their findings and make informed decisions based on the level of confidence they wish to achieve.
As we wrap up our exploration of statistical analysis with confidence, it is important to emphasize the practical applications of these techniques across various fields, from scientific research and business analytics to public policy and healthcare. The insights gained from statistical analysis can drive innovation, optimize processes, and inform strategic planning, leading to improved outcomes and informed decision-making.
Finally, I encourage readers to continue their learning journey in the realm of statistical analysis, as the field is vast and constantly evolving. By honing your statistical skills and deepening your understanding of key concepts and methodologies, you can unlock new opportunities for discovery and contribute meaningfully to the advancement of knowledge in your chosen domain.
In essence, statistical analysis with confidence is not just a technical practice but a mindset that empowers us to navigate the complexities of data, draw reliable conclusions, and make informed decisions that drive positive change. May this article serve as a stepping stone in your quest for statistical mastery and a deeper appreciation of the power of data-driven insights.